Deep Learning vs Machine Learning: Explore the key differences, benefits, and practical uses of AI to drive growth, scalability, and innovation for your business!
Introduction
You’ve heard the buzz—AI is transforming industries left and right, revolutionizing how businesses operate. But when it comes to deep learning vs machine learning, do you know what sets these two powerhouse technologies apart? If you’re a digital entrepreneur, understanding the difference isn’t just a tech curiosity—it’s a critical step toward leveraging AI to grow your business. While both are subsets of artificial intelligence, their unique capabilities can unlock new opportunities, whether you’re automating processes, analyzing data, or creating personalized customer experiences.
Imagine this: deep learning as the master of tackling complex, unstructured data like images, videos, and natural language, and machine learning as the go-to tool for crafting algorithms that excel at making daily decisions and predictions. Together, they hold the key to driving innovation and scaling your business like never before. In this post, we’ll break down these technologies, show you how they apply to your entrepreneurial goals, and help you determine which approach is right for your journey. Ready to dive in? Let’s decode AI hype and turn it into practical strategies you can use today.
Breaking Through the AI Hype: What Actually Works
If you’re running an online business, you’ve probably noticed the endless buzz around artificial intelligence. But beyond the hype and marketing speak, what does AI really mean for your bottom line in 2024? Let’s cut through the noise and focus on the AI tools and approaches that are actually delivering results.
Making AI Work for Your Business
At its core, AI is about automating repetitive tasks and improving predictions through data analysis. For example, you can use AI to personalize customer experiences by recommending relevant products, spot emerging market trends before competitors, or provide 24/7 customer service through chatbots. These practical applications directly impact efficiency and revenue.
Understanding AI Types: A Simple Comparison
Think of machine learning like a skilled carpenter – give them pre-cut wood pieces (structured data) and clear instructions (algorithms), and they’ll build high-quality furniture efficiently and reliably. The results are predictable and the process is straightforward. Distributed machine learning refers to the process of leveraging multiple computers or servers working together to handle and analyze massive datasets for machine learning purposes.
Deep learning is more like a master sculptor who can transform raw marble (unstructured data) into something extraordinary. Using an intuitive grasp of patterns (neural networks), they create unique and complex works. While this requires more resources, the results can be remarkable.
Deep Learning’s Sweet Spots
Deep learning excels at handling messy, unstructured data like images, text, and audio. If your business relies heavily on visual content, deep learning can automatically tag images, suggest personalized products, or flag suspicious activity. Tools like TensorFlow and PyTorch make these capabilities accessible to businesses of any size.
Machine Learning for Daily Operations
Machine learning works best with organized, structured data – think spreadsheets and databases. You can use it to predict which customers might leave, segment your audience for targeted marketing, or catch fraudulent transactions. This helps optimize operations and make smarter decisions based on data rather than gut feel.
Picking the Right AI Approach
Your choice between deep learning and machine learning depends on your specific needs. If you work with lots of unstructured data like images or text, deep learning may be ideal. For smaller datasets where you need clear, interpretable results, machine learning is often better. The key is matching the right tech solutions to your business goals while considering your data, technical capabilities, and budget. This practical approach helps ensure your AI investments deliver real value.
How Deep Learning Can Work for Your Business
Wondering how deep learning can practically benefit your online business ventures? You’re not alone. While this branch of machine learning may seem complex at first, it’s becoming increasingly accessible and valuable for businesses of all sizes. Let’s explore specific ways you can put deep learning to work for real business results.
Unlocking the Power of Unstructured Data
Deep learning excels at processing unstructured data like images, text, and audio – the kinds of messy, real-world information businesses deal with daily. For example, if you run an e-commerce store, deep learning can automatically analyze and tag product images to improve search functionality and help customers find what they’re looking for. It can also power smart recommendation engines that suggest products based on browsing history and preferences, leading to better conversion rates and happier customers.
Automating Customer Interactions and Support
Customer service is a prime area where deep learning can make an immediate impact. Modern chatbots powered by deep learning can handle complex customer inquiries 24/7, personalize responses based on context, and even proactively address issues before they escalate. This frees up your team to focus on higher-value work while ensuring customers get fast, helpful support whenever they need it.
Deep Learning in Market Analysis and Predictions
Deep learning can also give you a competitive edge in understanding markets and trends. By analyzing large datasets of customer reviews, social media posts, and market activity, deep learning algorithms uncover patterns that help you spot emerging opportunities and potential risks. This could mean predicting future product demand, identifying competitor strategies early, or fine-tuning marketing campaigns based on real customer feedback.
Getting Started with Deep Learning: Tools and Resources
While the underlying technology is sophisticated, several platforms now make deep learning accessible without requiring deep technical expertise. Frameworks like TensorFlow and PyTorch provide the building blocks for deep learning models, while cloud platforms like Google Cloud and Amazon Web Services offer pre-trained models and scalable infrastructure to help you get started quickly. This means you can begin experimenting with deep learning without needing a dedicated AI team.
Assessing Your Deep Learning Readiness
Before diving in, take time to assess if your business is ready for deep learning. These systems typically need significant data to train effectively. Consider: Do you have enough quality data? Do you have the computing resources to process it? Can you commit the time and effort needed to implement and maintain these systems? An honest evaluation will help you prioritize where deep learning can have the biggest impact. While deep learning offers exciting possibilities, it works best when matched to clear business needs and available resources. Taking a strategic approach ensures your investment in this technology drives real growth and innovation for your business.
Leveraging Machine Learning Without Getting Lost
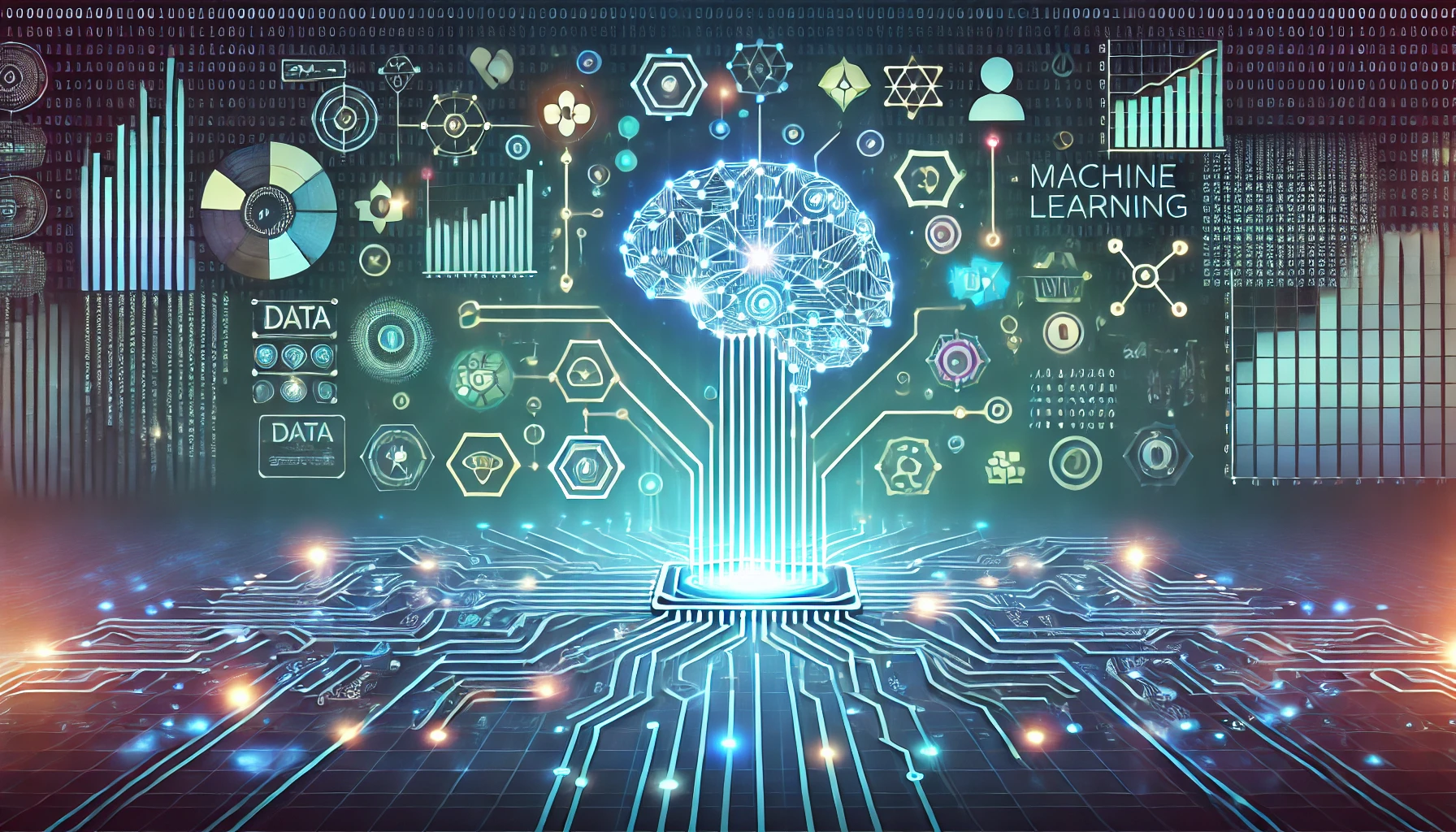
Machine Learning can seem overwhelming, but you don’t need complex systems or massive data sets to make it work for your business. Many entrepreneurs are finding success by focusing on practical applications and readily available tools. Let’s explore how you can use machine learning effectively while keeping things manageable.
Identifying Your Quick Wins with Machine Learning
Look at your daily operations with fresh eyes. Where do you spend time on repetitive tasks that could be automated? What data could help you make smarter decisions? These areas often present the best opportunities for machine learning to deliver immediate value. For example, if you manually segment customers for email campaigns, machine learning can automate this process and free up your time. Or if fraud detection is a concern for your e-commerce site, machine learning algorithms can flag suspicious transactions before they impact your revenue.
Choosing the Right Machine Learning Tools for the Job
You don’t have to build everything from scratch. Many user-friendly machine learning tools can help you get started quickly. If you use Google Cloud or Amazon Web Services, they offer pre-built models for tasks like predicting customer behavior, analyzing sentiment, and recommending products – all without requiring deep technical expertise. Another solid option is Azure Machine Learning Studio, which provides drag-and-drop tools for creating and deploying machine learning models.
Avoiding Common Machine Learning Pitfalls
Before jumping into any machine learning project, take time to define your goals clearly. Ask yourself: What specific problem am I trying to solve? What data do I have available? How will I measure success? This focused approach helps prevent wasted time and resources. Data quality assurance is also crucial – the old saying “garbage in, garbage out” definitely applies here. Make sure your data is clean, accurate and relevant through proper preparation and processing.
Learning From the Successes (and Mistakes) of Others
One of the best ways to learn is by studying how other businesses have used machine learning effectively. Look for case studies and success stories in your industry. What challenges did they face? What worked and what didn’t? Pay attention to both successes and failures – understanding what went wrong in unsuccessful projects can help you avoid similar issues. Was it poor data quality? Misalignment between the technology and business needs? Limited resources? These insights will give you a realistic view of what it takes to succeed with machine learning.
By staying practical, using the right data quality tools, and learning from others’ experiences, you can harness machine learning to grow your business sustainably. Remember, you don’t need to be an AI expert – start small, stay focused on real business problems, and build from there.
Building Your AI Decision Framework
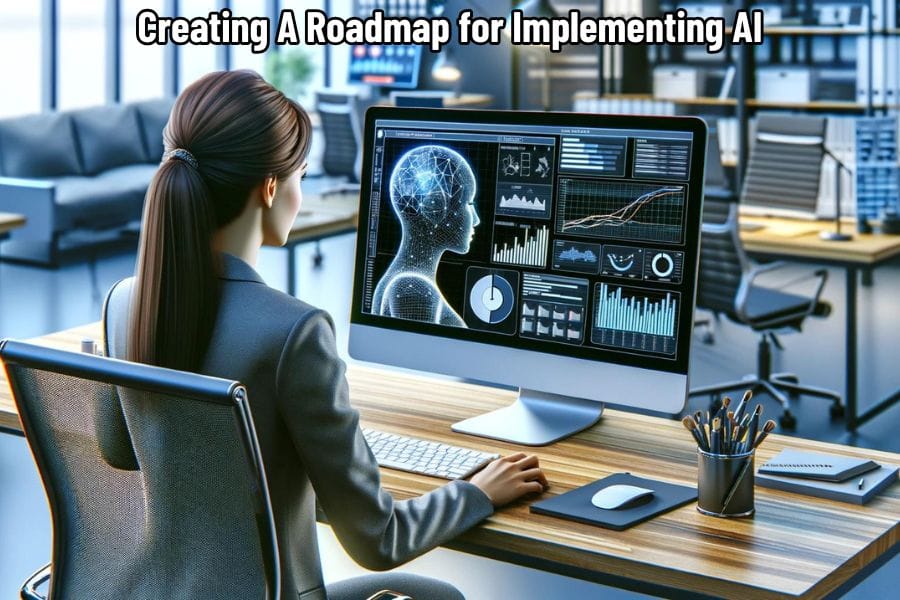
After exploring the key concepts, let’s focus on practical steps for integrating AI into your business. As an entrepreneur, choosing between deep learning and machine learning can feel daunting. This section provides a clear framework to help you select the AI solution that fits your specific business needs.
Assessing Your Business Needs and Objectives
Start by identifying the specific problems you want to solve. Are you looking to automate customer service, personalize product recommendations, or analyze market data? Having clear objectives helps determine which AI approach makes the most sense. For example, if you want to improve customer segmentation for targeted marketing, machine learning might be your best choice since it excels at handling structured data and provides clear insights you can act on to shape your marketing strategy.
Evaluating Your Data Landscape: Quality and Quantity
Your organizational data matters. Do you have large amounts of unstructured data like images and text, or smaller, organized datasets? This significantly impacts whether deep learning or machine learning will serve you better. Deep learning performs best with large volumes of unstructured data, while machine learning often works better with smaller, structured datasets where you need clear interpretability. Remember that data quality matters just as much as quantity – ensure your data is clean, accurate and relevant to your goals.
Considering Your Technical Capabilities and Resources
Assess your technical resources realistically. Do you have the expertise to build and maintain complex deep learning models in-house, or would a more accessible machine learning solution make more sense as a starting point? Deep learning typically requires significant computing power, including specialized GPUs. If you lack these resources, cloud platforms like Google Cloud and Amazon Web Services provide pre-trained models and scalable infrastructure to get you started. You might also consider user-friendly platforms like Azure Machine Learning Studio with its drag-and-drop interface if that better matches your current technical capabilities.
Building Your Implementation Roadmap
Once you’ve evaluated your needs, data, and resources, create a realistic roadmap for implementation. Your plan should outline your chosen AI approach, budget, timeline, and ways to measure success. Remember that implementing AI is an ongoing process. Start small with a focused project to gain experience and show value. From there, you can expand your AI initiatives as you build expertise. This measured approach reduces risk and lets you learn from early wins and challenges. For instance, if you want to automate customer service, you might begin with a basic rule-based chatbot before adding machine learning to personalize responses.
Making the Decision: A Practical Example
Consider this scenario: You run an e-commerce business with a large product image library. You want to improve product discovery and personalize recommendations. You have extensive image data but limited AI expertise in-house. In this case, using a pre-trained deep learning model through Google Cloud or AWS could work well as a first step. This would let you automatically tag images, analyze visual similarities, and power product recommendations without building complex models from scratch. As your business grows and your AI capabilities expand, you could then develop custom deep learning models to further enhance your product discovery system. This shows how your AI strategy can evolve alongside your business needs.
Scaling AI Without Breaking Your Business
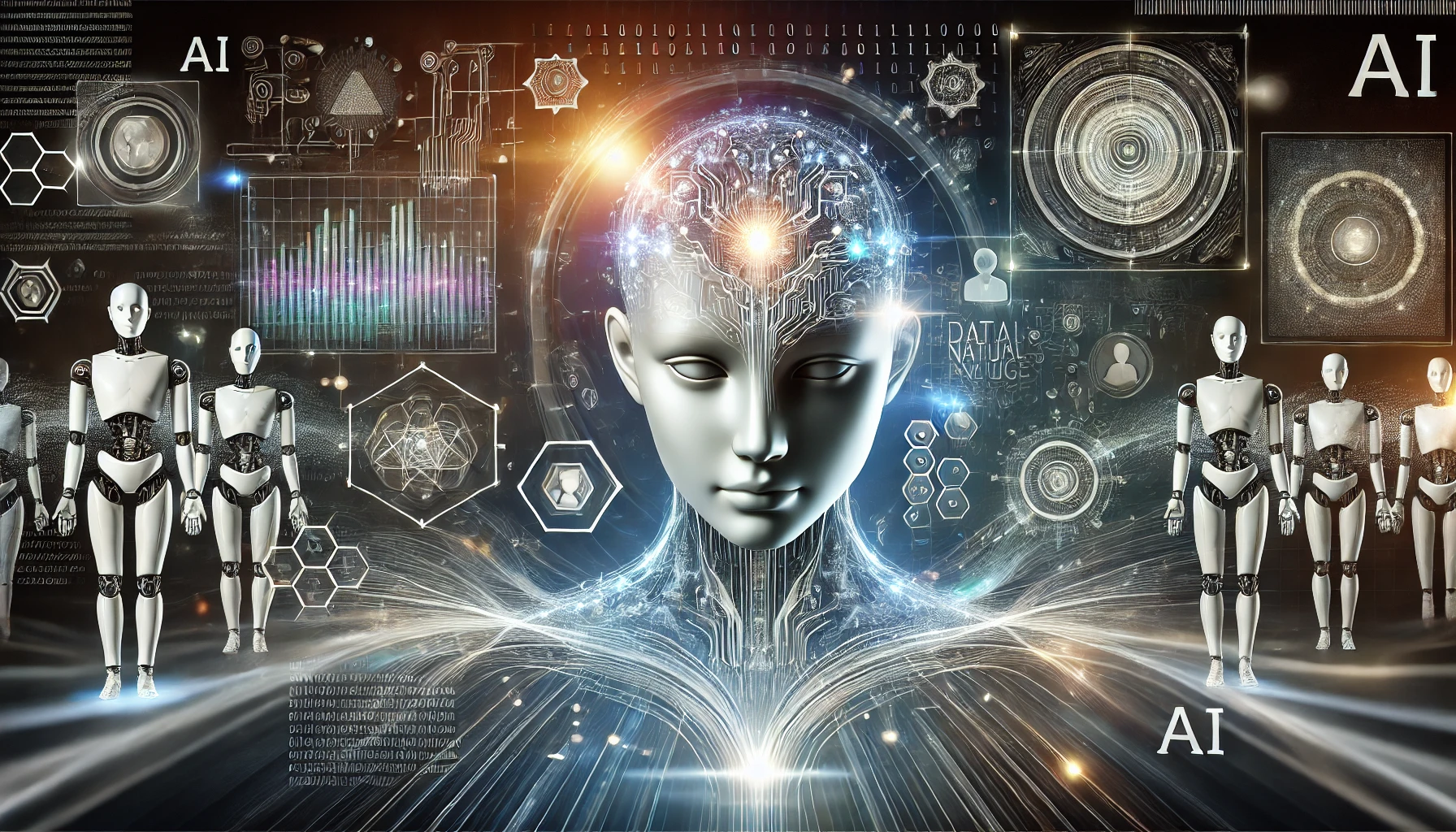
Looking to expand your business through AI but unsure where to start? Like many entrepreneurs, you’ve probably tested AI tools or experimented with machine learning. The real challenge comes in scaling these initial projects effectively while managing your resources. Let’s explore practical ways to grow your AI initiatives sustainably.
Building a Foundation for Scalable AI
Just as you wouldn’t build a house starting with the roof, scaling AI requires a strong foundation. Begin by ensuring your current AI projects deliver clear value and measurable results. This means defining specific goals, gathering quality data, and having at least one team member skilled in managing AI initiatives. For example, if you’ve used machine learning to personalize email campaigns, measure the impact through metrics like open rates and click-throughs. These concrete results show the value of your AI efforts and support further investment.
Growing Your AI Capabilities Sustainably
After proving success with pilot projects, you can thoughtfully expand your AI efforts. Rather than jumping into complex technologies, consider applying your existing machine learning approaches to new areas. You might use similar techniques to optimize pricing or improve how you segment customers. This measured approach helps you build expertise while learning what works best for your specific needs. Cloud platforms like Google Cloud or Amazon Web Services offer pre-trained models and flexible infrastructure, letting you experiment without major upfront costs.
Developing Your AI Team and Expertise
As your AI initiatives expand, focus on growing your team’s capabilities. This could mean training current staff, bringing in specialists, or working with outside experts. Building internal knowledge is key for long-term growth. Say you’re expanding your email personalization – you might need a data scientist to improve your models or an engineer to handle larger datasets. These targeted hires ensure you have the right expertise to manage growing AI systems.
Managing Costs and Maintaining Value
While scaling AI involves costs, focus on maximizing returns rather than just cutting expenses. Smart investments in infrastructure and tools often pay off over time. As you handle more data and complex models, moving to cloud services can be both cost-effective and scalable. Consider your future needs carefully – will you need specialized hardware for advanced AI models? Can you effectively manage your own infrastructure? These questions shape your scaling strategy. Remember that successful AI scaling requires constant monitoring and updates. Your data and needs will change, so your models must adapt to keep delivering value and supporting business growth.
Closing
As a digital entrepreneur, you now have a clearer picture of how deep learning and machine learning can transform your business. These technologies aren’t just buzzwords—they’re tools that can help you unlock insights, streamline operations, and elevate customer experiences. Deep learning shines when it comes to processing unstructured data and making predictions with incredible precision, while machine learning offers practical solutions for day-to-day challenges and decision-making. Armed with this knowledge, you’re better equipped to assess your business needs, evaluate your data landscape, and choose the AI approach that aligns with your goals.
The path forward is yours to define. Whether you start small with machine learning quick wins or dive into the innovative potential of deep learning, the opportunities are vast. Take the time to build a solid AI decision framework, invest in the right tools, and scale thoughtfully to ensure long-term success. AI is no longer a tool for just the tech giants—it’s a game-changer for entrepreneurs like you who are ready to innovate, grow, and lead. So, what’s your next move? The future of your business might just depend on the AI decisions you make today.
AI FAQs: Deep Learning vs Machine Learning
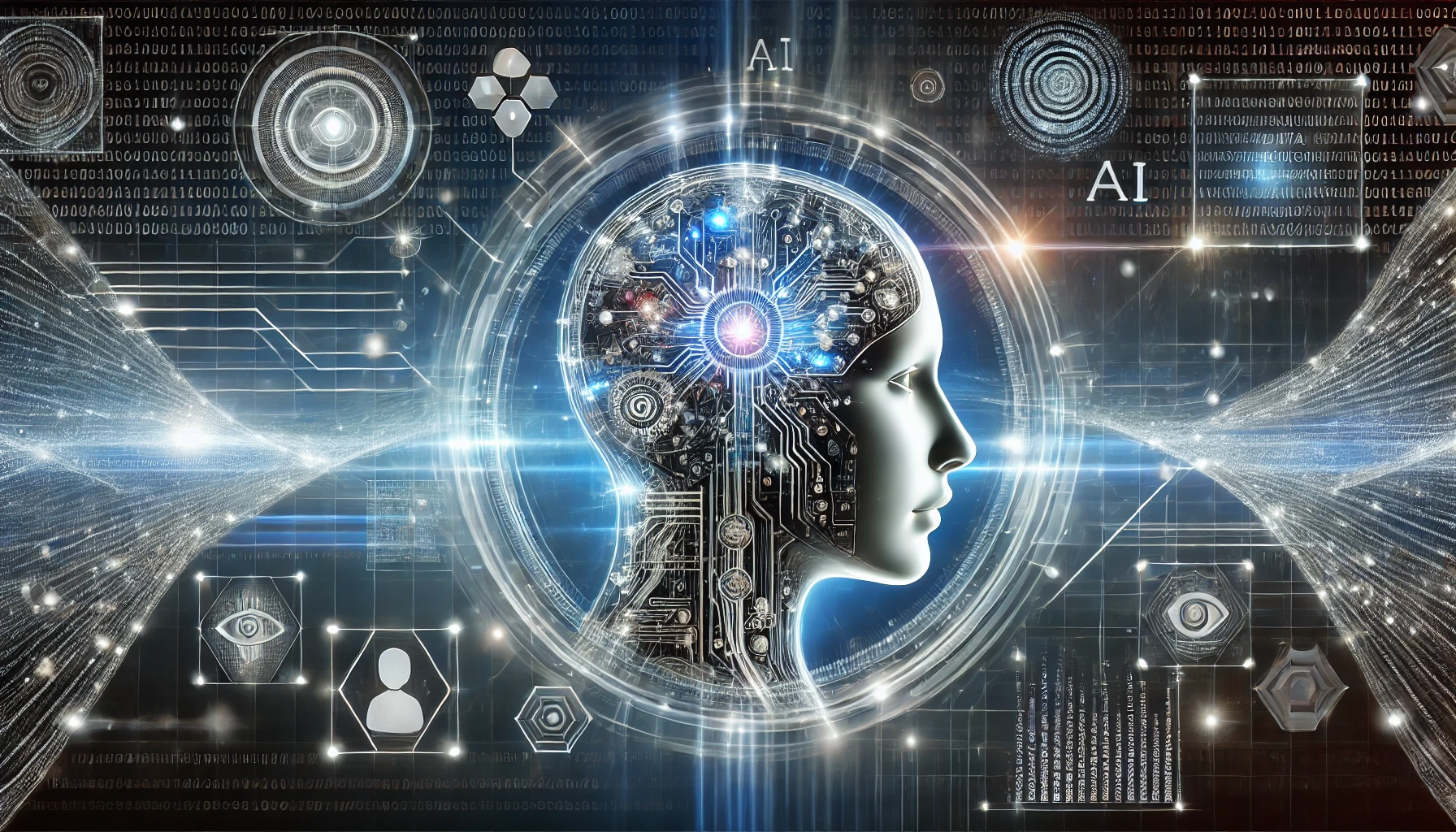
What are the main differences between deep learning and machine learning?
Answer: Deep Learning vs Machine Learning
Deep learning and machine learning are closely related, but they’re not the same. Machine learning focuses on creating algorithms that allow systems to learn from data and make predictions or decisions without being explicitly programmed. It often requires structured data and works well for tasks like recommendation systems or fraud detection. Deep learning, on the other hand, is a subset of machine learning that mimics the way the human brain processes information. It uses neural networks to analyze large, unstructured data sets like images, text, or audio. If machine learning is like teaching a student a set of rules, deep learning is like teaching them to think critically and solve problems creatively.
Why is deep learning important for digital entrepreneurs?
Answer: Deep Learning vs Machine Learning
As a digital entrepreneur, you’re likely working with vast amounts of unstructured data—social media interactions, customer reviews, and website traffic, for example. Deep learning excels at unlocking the insights hidden in this type of data. It can help you predict customer behavior, personalize marketing campaigns, and even automate customer support with intelligent chatbots. By leveraging deep learning, you’re not just keeping up with trends; you’re staying ahead of the curve, creating opportunities to innovate and grow your business.
Can small businesses benefit from machine learning?
Answer: Deep Learning vs Machine Learning
Absolutely. Machine learning isn’t reserved for big corporations with deep pockets. As a small business owner, you can use machine learning in manufacturing to automate repetitive tasks. You can use machine learning to enhance customer experiences, and optimize marketing efforts. For example, you could use it to analyze customer purchase patterns and recommend products, or to fine-tune your email campaigns based on engagement data. With affordable tools and platforms available, machine learning is more accessible than ever, allowing you to achieve big results without a massive investment.
How do I choose between deep learning and machine learning for my business?
Answer: Deep Learning vs Machine Learning
Start by assessing your business needs and the type of data you have. If your challenges involve structured data and straightforward decision-making, machine learning might be the best fit. It’s cost-effective and easier to implement. However, if you’re dealing with unstructured data like images, videos, or text, and you want to solve complex problems or predict trends with precision, deep learning could offer the most value. Consider your resources, technical expertise, and goals to decide which approach will drive the most impactful results.
What industries are most impacted by deep learning and machine learning?
Answer: Deep Learning vs Machine Learning
You’ll find machine learning and deep learning making waves in nearly every industry. In healthcare, they’re used for diagnostics and personalized treatments. In retail, they drive recommendation engines and dynamic pricing strategies. Advances in financial machine learning for fraud detection and risk assessment. Even creative industries like entertainment are using AI to analyze trends and create content. As a digital entrepreneur, this means no matter your niche, there’s likely a way these technologies can enhance your operations and offerings.
What are some challenges in implementing deep learning and machine learning?
Answer: Deep Learning vs Machine Learning
Implementing deep learning and machine learning isn’t without its hurdles. You may face challenges like insufficient data quality, lack of technical expertise, or high upfront costs. Additionally, scaling these technologies requires a robust infrastructure and ongoing maintenance. As you adopt AI, it’s crucial to manage expectations, start with smaller projects, and ensure your team has the right training and tools. The good news? Overcoming these challenges can lead to transformative benefits for your business, making the effort well worth it.
Attention Digital Entrepreneur’s, check out this All-In-One Digital Business Solution:
[JOIN HERE FOR FREE]