In today’s rapidly evolving digital landscape, the terms AI vs Machine Learning are often used synonymously, however, it is important to discern that machine learning constitutes just one aspect of the vast field of AI. These groundbreaking technologies hold immense potential to revolutionize numerous industries by automating processes, enhancing decision-making capabilities, and unlocking valuable insights from vast amounts of data. Understanding the distinctions and relationships between Artificial Intelligence (AI) and Machine Learning (ML) is crucial for organizations seeking to harness their power for unparalleled business success.
Short Summary
Before we dive into the details of AI vs machine learning, let’s clarify the definitions of AI and ML, and understand the relationship between the two technologies.
Definition of Artificial Intelligence (AI): AI refers to the development of intelligent systems that can perform tasks that typically require human intelligence. It involves simulating human-like intelligence in machines to enable them to solve problems, make decisions, and learn from data.
Definition of Machine Learning (ML): ML is a subset of AI that focuses on enabling machines to learn from data without being explicitly programmed. It involves developing algorithms that can analyze data, recognize patterns, and make predictions or decisions based on the insights gained.
While AI encompasses a broader scope of intelligent systems, ML plays a crucial role in enabling machines to learn and adapt through data analysis. They are intricately linked, with ML algorithms acting as the backbone of AI systems.
Understanding Artificial Intelligence (AI)

Explanation of AI and its Purpose
AI aims to replicate human intelligence by developing intelligent systems capable of performing complex tasks. These systems can analyze vast amounts of data, derive insights, and make decisions autonomously or with minimal human intervention. The purpose of AI is to enhance and automate various processes, improve efficiency, and drive innovation across industries.
Historical Background of AI
The concept of AI dates back several decades, with the first official AI conference held in 1956 at Dartmouth College. Over the years, AI has evolved tremendously, driven by advancements in computing power, data availability, and algorithmic breakthroughs. From early expert systems to modern deep learning techniques, AI in business has come a long way, revolutionizing industries and shaping our future.
Key Components and Techniques Used in AI
AI systems consist of several key components and techniques, including:
- Algorithms: Mathematical models or rules that enable machines to perform specific tasks.
- Deep Learning: A subset of ML that involves training artificial neural networks with multiple layers to process complex data and extract meaningful insights.
- Data Analysis: The process of inspecting, transforming, and modeling data to uncover patterns and insights.
- Predictive Modeling: Building models that make predictions or forecasts based on historical data.
- Neural Networks: Networks of interconnected artificial neurons that enable pattern recognition and data processing.
- Automation: Enabling systems to perform tasks autonomously without human intervention.
These components and techniques form the foundation of AI, enabling intelligent automation, predictive analytics, and smart decision-making in various domains.
Understanding Machine Learning (ML)

Explanation of ML and its Purpose
ML focuses on developing algorithms and models that allow machines to learn and improve from data without explicit programming. Its purpose is to enable machines to recognize patterns, make predictions, and make data-driven decisions based on the insights derived from the data.
Distinction between Supervised and Unsupervised Learning
In ML, there are two primary categories of learning: supervised learning and unsupervised learning.
- Supervised Learning: In supervised learning, the algorithm is trained using labeled data, where the desired output is known. The algorithm learns to map input data to specific outputs, making predictions or classifications on unseen data based on the learned patterns.
- Unsupervised Learning: On the other hand, unsupervised learning involves training the algorithm with unlabeled data. The algorithm learns patterns, structures, or relationships in the data without any predefined outcomes. Unsupervised learning is often used for exploratory data analysis and identifying hidden patterns in the absence of labeled data.
Overview of ML Algorithms and Models
ML algorithms and models are the driving force behind the learning and predictive capabilities of ML systems. Some common ML algorithms and models include:
- Decision Trees: Hierarchical tree-like structures that make decisions based on a sequence of questions or conditions.
- Regression: Techniques that analyze the relationship between dependent and independent variables to make predictions or estimate values.
- Clustering: Algorithms that group similar data points together to identify patterns or relationships.
- Neural Networks: Networks of artificial neurons that are trained to process data and make predictions.
- Reinforcement Learning: Learning through trial and error, where an agent interacts with an environment to maximize rewards.
These algorithms and models enable ML systems to analyze data, make predictions, and uncover valuable insights.
AI vs Machine Learning: Differences and Similarities

Defining the Boundaries between AI and ML
The boundaries between AI vs machine learning are often blurred, as ML is an essential component within the broader field of AI. AI encompasses a wide range of intelligent systems that may or may not involve ML techniques. ML, on the other hand, focuses specifically on enabling machines to learn from data.
Key Differences in the Approaches and Goals of AI and ML
While AI and ML overlap in many areas, they differ in their approaches and goals:
- AI aims to develop intelligent systems capable of mimicking human-like intelligence and performing complex tasks without explicit programming.
- ML focuses on developing algorithms that allow machines to learn from data and make predictions or decisions based on the learned patterns.
In summary, AI is a broader field, encompassing various approaches and techniques, while ML is a subset of AI, focusing primarily on data-driven learning and decision-making.
Overlapping Concepts and Techniques in AI and ML
AI and ML share several concepts and techniques, including:
- Pattern Recognition: Both AI and ML involve recognizing patterns and extracting meaningful insights from data.
- Data Analysis: Both fields utilize data analysis techniques to gain insights and make informed decisions.
- Automation: AI and ML enable automation, allowing systems to perform tasks autonomously.
These overlapping concepts and techniques contribute to the synergy between AI and ML, driving advancements and enabling intelligent automation.
Applications of Artificial Intelligence

Real-Life Examples of AI Applications in Various Industries
AI has found applications in numerous industries, revolutionizing the way businesses operate. Here are some real-life examples:
- Healthcare: AI is used for medical image analysis, disease diagnosis, personalized treatment recommendations, and drug discovery.
- Finance: AI powers fraud detection systems, credit scoring models, algorithmic trading, and personalized financial advice.
- Retail: AI enables personalized recommendations, demand forecasting, inventory management, and customer service chatbots.
- Transportation: AI is utilized in self-driving cars, traffic management systems, route optimization, and predictive maintenance.
- Manufacturing: AI improves quality control, predictive maintenance, supply chain optimization, and autonomous robots.
Benefits and Challenges of Implementing AI Systems
The implementation of AI systems brings various benefits, including:
- Improved Efficiency: AI automates processes, reduces human errors, and enhances productivity.
- Enhanced Decision-Making: AI systems analyze vast amounts of data quickly, enabling more informed and data-driven decision-making.
- Increased Innovation: AI fosters innovation by enabling the development of new products, services, and business models.
However, implementing AI systems also presents challenges, such as:
- Data Privacy and Security: AI systems rely on access to large amounts of data, raising concerns about data privacy and security.
- Ethical Considerations: The potential impact of AI on society, job displacement, and biased decision-making raises ethical concerns that need to be addressed.
- Regulatory Compliance: AI implementation requires compliance with various regulations and standards to ensure ethical and responsible use.
Potential Future Developments in AI Technology
The future of AI holds immense potential for further advancements. Some potential developments include:
- Automated Learning: Continued progress in AI research will lead to the development of systems that can learn autonomously from limited or no human supervision.
- AI Algorithms: Advancements in AI algorithms will enable more accurate predictions, better decision-making, and improved performance of AI systems.
- Predictive Analytics: AI will continue to enhance predictive analytics capabilities, leading to more accurate forecasts and smarter insights.
- Smart Machines: The integration of AI in various devices and machines will lead to the development of smarter, more autonomous systems.
- Cognitive Computing: AI systems will become more capable of interpreting and understanding human language, leading to significant advancements in natural language processing.
The future of AI is promising, with countless opportunities for businesses to leverage its capabilities and drive innovation across industries.
Applications of Machine Learning

Real-Life Examples of ML Applications in Different Domains
ML has transformed various domains with its powerful predictive capabilities. Here are some real-life examples:
- E-commerce: ML powers personalized product recommendations, customer segmentation, and fraud detection systems.
- Healthcare: ML aids in disease diagnosis, patient monitoring, drug discovery, and precision medicine.
- Marketing: ML enables targeted advertising, customer behavior analysis, sentiment analysis, and sales forecasting.
- Banking: ML assists in credit scoring, risk assessment, fraud detection, and anomaly detection.
- Energy: ML optimizes energy consumption, predicts equipment failures, and facilitates smart grid management.
Benefits and Challenges of Implementing ML Systems
Implementing ML systems comes with numerous benefits:
- Improved Predictive Capabilities: ML systems make accurate predictions based on insights derived from historical data.
- Enhanced Decision-Making: ML assists in data-driven decision-making, enabling businesses to gain a competitive edge.
- Cost and Time Savings: ML automates tasks, reducing manual effort and increasing operational efficiency.
However, implementing ML systems also poses challenges such as:
- Data Quality and Quantity: ML models rely on high-quality, diverse, and sizable datasets for optimal performance.
- Model Interpretability: Complex ML models often lack transparency, making it challenging to interpret their decision-making process.
- Implementation Complexity: Developing and deploying ML models requires expertise in data engineering, algorithm selection, and infrastructure.
Potential Future Developments in ML Technology
The future of ML holds immense possibilities for advancements and innovations. Some potential developments include:
- Statistical Modeling: Advancements in statistical modeling techniques will lead to more accurate predictions and improved insights.
- Supervised Learning: ML algorithms will continue to evolve, enabling improved predictions and classifications.
- Unsupervised Learning: Techniques for unsupervised learning will become more sophisticated, uncovering hidden patterns and relationships in data.
- Reinforcement Learning: Further research in reinforcement learning will enable machines to make optimal decisions in complex environments.
- Interdisciplinary Applications: ML will continue to find applications in diverse fields, such as healthcare, finance, and climate modeling.
The future of ML is bright, with tremendous potential for advancements that will further revolutionize industries and drive innovation.
Relationship between AI and Machine Learning

How ML Facilitates AI Development and Capabilities
ML plays a crucial role in facilitating AI development and enhancing its capabilities. ML enables AI systems to learn and adapt through data analysis, uncovering patterns, and making predictions. By applying ML algorithms to large volumes of data, AI systems can improve their decision-making and intelligence over time.
Role of ML Algorithms in Training AI Systems
The success of AI systems heavily relies on the quality and performance of ML algorithms. ML algorithms provide the necessary framework for training AI systems, allowing them to process and learn from massive amounts of data. By selecting appropriate ML algorithms and models, AI systems can acquire the intelligence required to perform complex tasks.
Mutual Influence and Synergy between AI and ML
The relationship between AI vs machine learning is characterized by mutual influence and synergy. AI leverages ML techniques to enhance its learning and decision-making capabilities, while ML relies on AI systems for applying learned patterns and making intelligent predictions. This mutual influence leads to continuous advancements in both fields, driving innovation and shaping the future of intelligent automation.
The Future of AI and Machine Learning
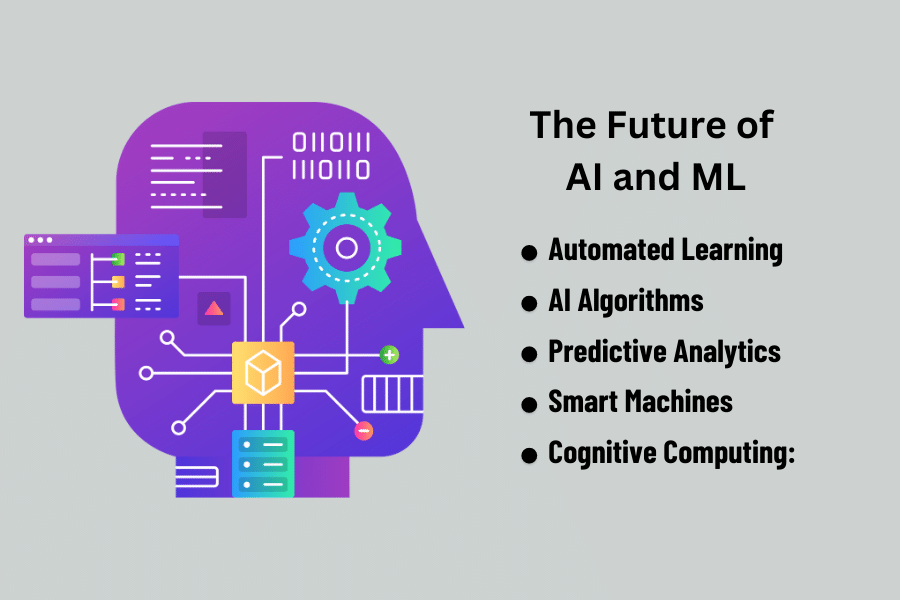
Predictions and Trends for AI Advancements
The future of AI holds exciting possibilities:
- Automated Learning: AI systems will become increasingly capable of learning autonomously from limited or no human supervision.
- AI Algorithms: Advancements in AI algorithms will lead to more accurate predictions, smarter decision-making, and improved performance of AI systems.
- Predictive Analytics: AI will continue to enhance predictive analytics capabilities, enabling more accurate forecasts and smarter insights.
- Smart Machines: The integration of AI in various devices and machines will lead to the development of smarter, more autonomous systems.
- Cognitive Computing: AI systems will become more capable of interpreting and understanding human language, leading to significant advancements in natural language processing.
Conclusion
Recap of the Main Differences and Similarities Between AI and ML
In summary, Artificial Intelligence (AI) is the broader field focused on creating intelligent systems, while Machine Learning (ML) is a subset of AI that enables machines to learn and improve from experience without being explicitly programmed. AI encompasses the development of intelligent systems, whereas ML provides the algorithms and techniques necessary for learning and performance improvement.
Importance of AI and ML in Shaping Our Future
AI and ML are transforming industries and revolutionizing how we live and work. Their potential to automate tasks, optimize processes, and extract insights from data is unparalleled. By leveraging the power of AI and ML, organizations can gain a competitive edge, unlock new revenue streams, and drive unprecedented business success.
Encouragement for Continued Exploration and Development in These Fields
As AI and ML continue to evolve, it is crucial to encourage exploration, research, and development in these fields. By pushing the boundaries of AI and ML, we can unlock new possibilities, solve complex problems, and shape a future where intelligent technologies enhance the lives of individuals and drive remarkable societal advancements.
AI vs Machine Learning: Frequently Asked Questions
What is the difference between AI vs machine learning?
AI is the broader field focused on creating intelligent systems, while ML is a subset of AI that enables machines to learn and improve from experience without being explicitly programmed.
What are some real-life applications of Artificial Intelligence (AI) in various industries?
AI is utilized in healthcare for medical image analysis, finance for fraud detection, retail for recommendation systems, manufacturing for predictive maintenance, and transportation for autonomous vehicles, among many others.
Q3: What are the benefits of implementing Machine Learning (ML) systems?
ML systems enhance decision-making, automate tasks, improve customer experiences, and provide data-driven insights, leading to increased efficiency and productivity.
How do Machine Learning (ML) algorithms facilitate the training of AI systems?
ML algorithms such as neural networks, decision trees, and support vector machines enable AI systems to learn from data, recognize patterns, make predictions, and improve their performance over time.
What is the future of AI and ML?
The future of AI includes enhanced natural language processing, contextual awareness, and explainable AI, while ML advancements will impact AI’s capabilities, enabling it to solve increasingly complex problems and drive innovation.
What ethical considerations are associated with AI and ML?
Ethical considerations include addressing biases in AI algorithms, ensuring transparency and accountability, and addressing the potential impact of AI and ML on employment and society.
How can organizations harness the power of AI and ML for unrivaled business success?
Organizations can leverage AI and ML to automate processes, make data-driven decisions, enhance customer experiences, and unlock new revenue opportunities, ultimately leading to unparalleled business success.
FREE AI POWERED SOFTWARE FOR DIGITAL BUSINESSES: [CLICK HERE TO LEARN MORE]